Artificial Intelligence Models to Predict Disability for Mental Health Disorders
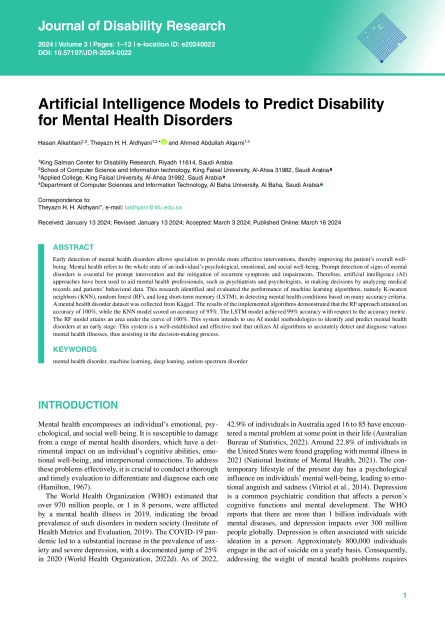
- Hasan Alkahtani
- Theyazn H Aldhyani
- Ahmed Abdullah Alqarni
Journal of Disability Research
10.57197/JDR-2024-0022Abstract
Early detection of mental health disorders allows specialists to provide more effective interventions, thereby improving the patient’s overall well-being. Mental health refers to the whole state of an individual’s psychological, emotional, and social well-being. Prompt detection of signs of mental disorders is essential for prompt intervention and the mitigation of recurrent symptoms and impairments. Therefore, artificial intelligence (AI) approaches have been used to aid mental health professionals, such as psychiatrists and psychologists, in making decisions by analyzing medical records and patients’ behavioral data. This research identified and evaluated the performance of machine learning algorithms, namely K-nearest neighbors (KNN), random forest (RF), and long short-term memory (LSTM), in detecting mental health conditions based on many accuracy criteria. A mental health disorder dataset was collected from Kaggel. The results of the implemented algorithms demonstrated that the RF approach attained an accuracy of 100%, while the KNN model scored an accuracy of 95%. The LSTM model achieved 99% accuracy with respect to the accuracy metric. The RF model attains an area under the curve of 100%. This system intends to use AI model methodologies to identify and predict mental health disorders at an early stage. This system is a well-established and effective tool that utilizes AI algorithms to accurately detect and diagnose various mental health illnesses, thus assisting in the decision-making process.
Keywords
mental health disorder, machine learning, deep leaning, autism spectrum disorderCitation
Alkahtani, H., Aldhyani, T.H., & Alqarni, A.A. (). Artificial Intelligence Models to Predict Disability for Mental Health Disorders. Journal of Disability Research, 3(3), doi: 10.57197/JDR-2024-0022
Link to this page: https://res.adhd.org.sa/doi/10.57197/JDR-2024-0022